Marketing's Biggest Problem
Marketing is an inefficient practice.
Most marketers operate under false assumptions and anecdotal knowledge.
(Marketing) 'best practices' is still dominated by impressions and untested assumptions.
The amount of marketing dollars wasted each year is hardly measurable. But it's in the order of billions of dollars. This makes measuring and maximizing marketing effectiveness an important concern for every reasonable Marketing department.
This is where data and measurements come in handy. Can we leverage data to enhance the effectiveness of marketing activities? Short answer: YES. If you want to know how, read on.
Let's Define Marketing Mix Modeling
Originally developed by econometricians in the 1950s and 60s (See Wikipedia), Marketing Mix Modeling (MMM) is an early example of the application of data within marketing. Today, MMM constitutes an integral component in every marketing department striving to become data-driven.
Generally speaking, MMM is about developing an analytical framework for efficient marketing resource allocation. Resource allocation varies within three key areas: channels, markets (regions), and time. More on that in future posts.
Before we proceed further, let's establish a few things.
Firstly, the sum of all allocations cannot exceed the total size of the marketing budget. This is the first and most essential constraint. There are, however, other types of constraints. For example, the minimum required allocation in a specific market or channel.
Also, the word efficient is vague. What makes an allocation efficient? Why is an allocation ineffective (AKA leading to budget leakage)?
The definition of efficient allocation is subjective and brand-specific. In practice, KPIs of interest define efficiency. Revenue is the most important KPIs. After all, who doesn't like more revenue? But, there are other important KPIs, especially long-term ones, such as brand awareness. Therefore, an efficient allocation must reflect the brand's objectives and strategy.
In summary, when implemented well, MMM will help marketing managers reduce marketing inefficiency and, therefore, less budget leakage. They can leverage internal data to improve performance and consistently deliver goals.
Common Pitfalls with MMM
MMM is about precisely predicting marketing outcomes and maximizing the ROI of marketing.
This is wrong!
Let's be honest: most modelers and econometricians claim that their models can precisely predict outcomes and recommend the best marketing mix that delivers maximum growth. If achieving growth was that simple, every marketing manager should've been out of business.
Markets are inherently unpredictable
What most modelers care about is minimizing mean square error or model error. They use historical data and cross-validation to measure and minimize error. But things could easily go wrong when modeling complex systems such as markets.
The imaginary tale of Dr. James, head of Analytics
It's a lovely spring day in 2019, and you're sitting in a sleek NYC office of the marketing department of an international hotel conglomerate. The head of Analytics, Dr. James is presenting the latest MMM recommendations for 2020 marketing budget planning.
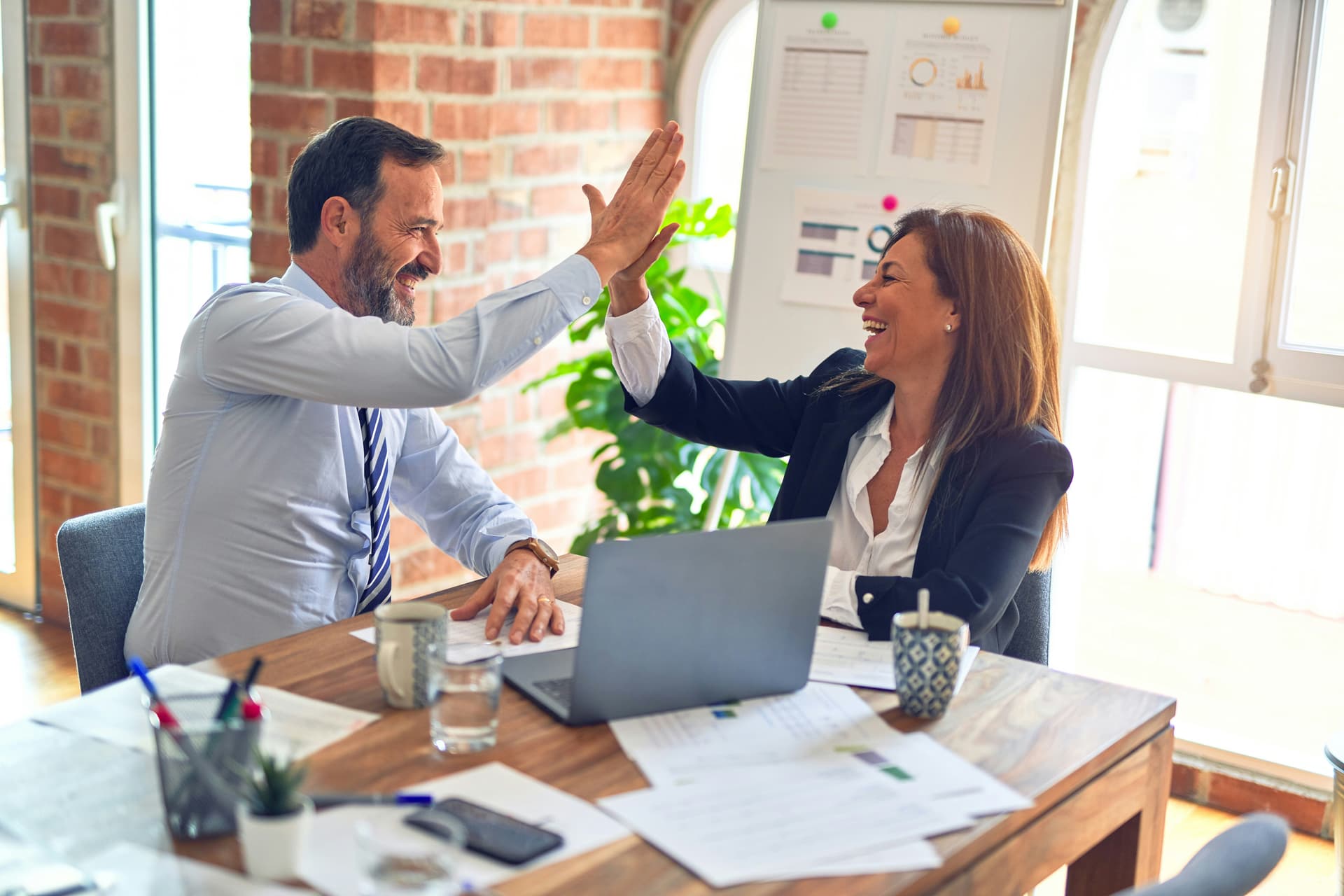
There is an air of confidence and optimism. All numbers point to a solid growth YoY over the past five years.
Since Dr. James joined in 2015, he and his talented team have delivered startling work. His team comprises several PhDs who received degrees from Yale and Princeton in Particle Physics, Genomics, and other esoteric topics.
The biggest deliverable of the Analytics team is a sophisticated MMM model with a prediction accuracy of %99.8! The model uses advanced techniques like Monte Carlo Sampling and Hierarchical Bayesian Regression. Thanks to the MMM model, Dr. James brags, we outperformed the business targets by +5 ppt (In other words, $1bn of additional revenues is attributed to the MMM recommendations).
Looking forward to the 2020s travel industry, the MMM seems bullish. Presenting the latest results, Dr. James gives several scientific evidence favoring budget increment. Mrs. Forester, Global Head of Growth, is happily listening. More marketing budget is exactly what she was asking the CMO and CFO.
The MMM recommends massive investments in billboards, TV ads, and influencers. It recommends spending most of the budget in Europe and the US in the summer of 2020. Sounds like a good plan. Except that it's not.
I let you think about the rest of the story yourself.
Stop using MMM for forecasting
The imaginary tale of the Hotel business above and Dr. James is the reality of many firms worldwide. So, if the MMMs are so wrong, why should we care?
Before throwing the baby out with the bathwater, listen to this. The MMM's goal is not to predict or forecast. Stop seeing them as the oracle to see into the future.
In short, MMM is not made to reduce uncertainty. So stop using it for prediction.
Bayesian Statistics is a framework to combine prior knowledge with new observations to update subjective belief. The goal of Bayesian is not to overcome uncertainty but to quantify the uncertainty. The uncertainty is very stubborn, and it doesn't simply go away.
Right Strategy for MMM
The MMM should be seen as a framework for combining various sources of information to understand marketing inefficiencies (owing to market uncertainty). The information varies from truths (hard data), half-truths (market and consumer research), and hopes or dreams (business targets).
Within this framework, people, data, and processes are unified. The Implicit becomes explicit. Actors are aligned with the invisible thread of data. Finally, marketing channels and activities are systemically evaluated.
A good CMO or Marketing VP understands the true philosophy of MMM. They start with a simple in-house MMM framework, align all the actors and stakeholders (i.e., cultural component), and gradually evolve the MMM framework to reflect the realities and challenges of their particular business.
Still remember, that doesn't mean we have overcome the marketing inefficiency or budget bleeding. But we have overcome the misalignments and chaotic execution—a first and most important step toward more effective marketing.
Finally, I'd like to remind everyone of George Box's golden phrase:
All models are wrong, but some are useful.
George Box